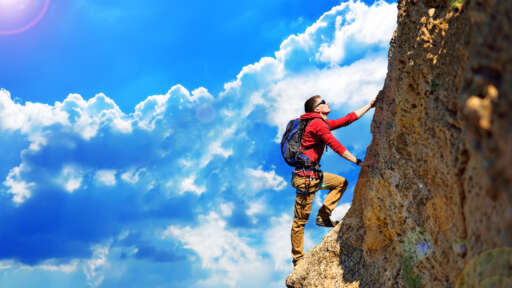
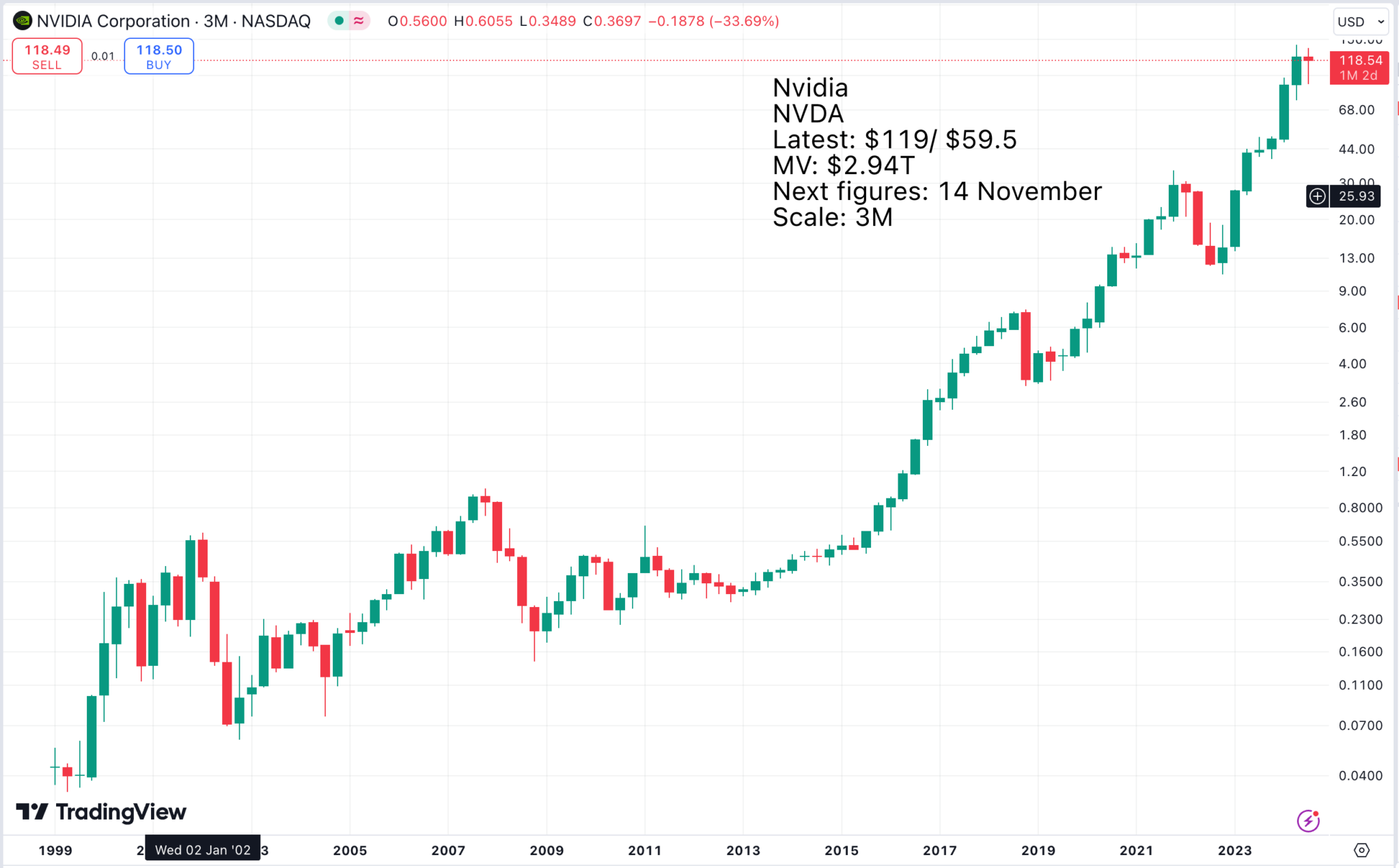
I have no idea what the immediate future holds for the Nvidia share price but it is impossible to read the transcript of the latest meeting with analysts and not be excited about prospects in anything but the very short-term.
Table of Contents
Unbelievable Numbers
Revenue of $30bn was up 15pc sequentially and up 122pc year on year and well above our outlook of $28bn. Starting with Data Centre.
Data Centre revenue of $26.3bn was a record, up 16pc sequentially and up 154pc year on year, driven by strong demand for NVIDIA Hopper, GPU computing, and our networking platforms. Compute revenue grew more than 2.5x. Networking revenue grew more than 2x from the last year. Cloud service providers represented roughly 45pc of our Data Centre revenue, and more than 50pc stemmed from the consumer Internet and enterprise companies.
Customers continue to accelerate their Hopper architecture purchases while gearing up to adopt Blackwell. Key workloads driving our Data Center growth include generative AI model training and inferencing; video, image, and text data pre and post processing with CUDA and AI workloads; synthetic data generation; AI-powered recommender systems; SQL and Vector database processing as well. Next-generation models will require 10 to 20 times more compute to train with significantly more data. The trend is expected to continue.
Colette Kress, CFO, Nvidia, Q2 2025, 28 August 2024
Sales at Nvidia, which has a habit of beating expectations, are expected to top $203bn for the year to 31 January 2027. This compares with £26.9bn for the year to 31 January 2022. Has there ever been a big company that has grown so fast?
The shares have risen dramatically but so they should have done. Another thing about Nvidia is that it is incredibly profitable. The net profit margin is over 50pc and the free cash flow margin (free cash flow as a percentage of revenue) is close to 50pc.
25pc of Revenue Feeds Back to Shareholders
The company is focused on looking after shareholders.
Cash flow from operations was $14.5bn. In Q2, we utilized cash of $7.4bn toward shareholder returns in the form of share repurchases and cash dividends, reflecting the increase in dividend per shareholder.
Our board of directors recently approved a $50bn share repurchase authorization to add to our remaining $7.5bn of authorization at the end of Q2.
Colette Kress, CFO, Nvidia, Q2 2025, 28 August 2024
My impression from these numbers is that Nvidia is using 25pc of revenue for dividends and share buybacks. The shares are marking time having sold off after the results but I can’t see them falling for long.
NVidia has an asset-light business. Its biggest cost is its employees (working for Nvidia is like winning the lottery). The company has around 30,000 employees, most of whom are serious white-collar types engaged in research and development or sales and marketing.
Momentum of Generative AI Accelerating
In answer to a question, Jensen Huang had this to say. The question was:
As you may know, there’s a pretty heated debate in the market on your customers and customers’ customers return on investment and what that means for the sustainability of capex going forward.
Internally at NVIDIA, like what are you guys watching? What’s on your dashboard as you try to gauge customer return and how that impacts capex?
Toshiya Hari, analyst, Nvidia Q2 2025 analysts’ meeting, 28 August 2024
Jensen Huang’s reply is below.
On the longer-term question, let’s take a step back. And you’ve heard me say that we’re going through two simultaneous platform transitions at the same time.
The first one is transitioning from general-purpose computing to accelerated computing. And the reason for that is because CPU scaling has been known to be slowing for some time and it has slowed to a crawl. And yet the amount of computing demand continues to grow quite significantly. You could maybe even estimate it to be doubling every single year.
And so, if we don’t have a new approach, computing inflation would be driving up the cost for every company, and it would be driving up the energy consumption of data centres around the world. In fact, you’re seeing that. And so, the answer is accelerated computing. We know that accelerated computing, of course, speeds up applications.
It also enables you to do computing at a much larger scale, for example, scientific simulations or database processing, but what that translates directly to is lower cost and lower energy consumed. And in fact, this week, there’s a blog that came out that talked about a whole bunch of new libraries that we offer. And that’s really the core of the first platform transition, going from general-purpose computing to accelerated computing. And it’s not unusual to see someone save 90pc of their computing cost.
And the reason for that is, of course, you just sped up an application 50x. You would expect the computing cost to decline quite significantly. The second was enabled by accelerated computing because we drove down the cost of training large language models or training deep learning so incredibly that it is now possible to have gigantic scale models, multitrillion-parameter models and train it on — pretrain it on just about the world’s knowledge corpus and let the model go figure out how to understand human language representation and how to codify knowledge into its neural networks and how to learn reasoning, and so which caused the generative AI revolution. Now, generative AI, taking a step back about why it is that we went so deeply into it is because it’s not just a feature, it’s not just the capability.
It’s a fundamental new way of doing software. Instead of human-engineered algorithms, we now have data. We tell the AI, we tell the model, we tell the computer what are the expected answers. What are our previous observations? And then for it to figure out what the algorithm is, what’s the function.
It learns a universal — AI is a bit of a universal function approximator and it learns the function. And so, you could learn the function of almost anything. And anything that you have that’s predictable, anything that has structure, anything that you have previous examples of. And so, now here we are with generative AI.
It’s a fundamental new form of computer science. It’s affecting how every layer of computing is done from CPU to GPU, from human-engineered algorithms to machine-learned algorithms, and the type of applications you could now develop and produce is fundamentally remarkable. And there are several things that are happening in generative AI. So, the first thing that’s happening is the frontier models are growing in quite substantial scale.
And they’re still seeing — we’re still all seeing the benefits of scaling. And whenever you double the size of a model, you also have to more than double the size of the data set to go train it. And so, the amount of flops [FLOPS measures the number of floating-point calculations/ operations that can be performed in one second] necessary in order to create that model goes up quadratically. And so, it’s not unexpected to see that the next-generation models could take 10, 20, 40 times more compute than last generation.
So, we have to continue to drive the generational performance up so we can drive down the energy consumed and drive down the cost necessary to do it. And so, the first one is there are larger frontier models trained on more modalities. And surprisingly, there are more frontier model makers than last year. And so, you have more and more and more.
That’s one of the dynamics going on in generative AI. The second is although it’s below the tip of the iceberg, what we see are ChatGPT image generators. We see coding. We use generative AI for coding quite extensively here at NVIDIA now.
We, of course, have a lot of digital designers and things like that. But those are kind of the tip of the iceberg. What’s below the iceberg are the largest systems, largest computing systems in the world today, which are — and you’ve heard me talk about this in the past, which are recommender systems moving from CPUs. It’s now moving from CPUs to generative AI.
So, recommender systems, ad generation, custom ad generation targeting ads at very large scale and quite hyper-targeting, search, and user-generated content, these are all very large-scale applications have now evolved to generative AI. Of course, the number of generative AI start-ups is generating tens of billions of dollars of cloud renting opportunities for our cloud partners. And sovereign AI, countries that are now realizing that their data is their natural and national resource and they have to use AI, build their own AI infrastructure so that they could have their own digital intelligence. Enterprise AI, as Colette mentioned earlier, is starting, and you might have seen our announcement that the world’s leading IT companies are joining us to take the NVIDIA AI Enterprise platform to the world’s enterprises.
The companies that we’re talking to, so many of them are just so incredibly excited to drive more productivity out of the company. And then general robotics. The big transformation last year as we are able to now learn physical AI from watching video and human demonstration and synthetic data generation from reinforcement learning from systems like Omniverse, we are now able to work with just about every robotics companies now to start thinking about, start building general robotics. And so, you can see that there are just so many different directions that generative AI is going.
And so, we’re actually seeing the momentum of generative AI accelerating.
Jensen Huang, CEO, Nvidia, Q2 2025, 28 August 2024
The simple conclusion is that Nvidia is the most amazing company ever, playing a critical role as the technology revolution goes ballistic.
Share Recommendations
Nvidia. NVDA. (30 August)
Strategy – Keep Taking the Pills, Buy Nvidia
Shares don’t always go up and many investors have done well from Nvidia. This means many investors are itching to take profits. Eventually, the selling will dry up and the shares will resume climbing. Keep the faith.